High-Resolution Guided Image Synthesis Using Latent Diffusion Model Technology
Keywords:
Ultrasound Images, diffusion models, Image Synthesis, reverse diffusion, Forward processAbstract
We introduce the latent diffusion model used in medical ultrasound image synthesis. We point out that precision is the issue, and installing ultrasound images was completed with an accuracy of 97.47% since ultrasound demands greater accuracy. It has some particular disadvantages because it operates in real-time and requires operator settings. Considering these challenges, our model has a lot of promise to provide accurate and lifelike ultrasound images. Even though it is hard to calculate the precise answer for this optimization, applying the backpropagation method merely once can produce an approximation. In order to train a diffusion model with the value and outcomes (FID: 2.870, CLIP: 0.209, SSIM: 0.9923, and LPIPS: 0.92) that we promised, we generated synthetic images of roughly 300 ultrasound images. were acquired. Expanding the use of artificial intelligence in medical imaging is the aim of this endeavor. Since this is a novel problem, the study will serve as a foundation and source of inspiration for researchers looking into possible applications of diffusion models in medical image production. The URL https://www.kaggle.com/datasets/suraahmed56/computer-vision-medical-images provides access to synthetic images
Downloads
References
Z. Jin, X. Shen, B. Li, and X. Xue, “Training-free Diffusion Model Adaptation for Variable- Sized Text-to-Image Synthesis,” no. NeurIPS, pp. 1–14, 2023, [Online]. Available: http://arxiv.org/abs/2306.08645
Z. Chen et al., “Hierarchical Integration Diffusion Model for Realistic Image Deblurring,”no. NeurIPS, pp. 1–12, 2023, DOI: http://arxiv.org/abs/2305.12966
J. Singh, S. Gould, and L. Zheng, “High-Fidelity Guided Image Synthesis with Latent Diffusion Models,” no. Lli, pp. 5997–6006, 2023, doi: 10.1109/cvpr52729.2023.00581.
L. Sun, J. Chen, Y. Xu, M. Gong, K. Yu, and K. Batmanghelich, “Hierarchical Amortized GAN for 3D High Resolution Medical Image Synthesis,” IEEE J. Biomed. Heal. Informatics, vol. 26, no. 8, pp. 3966–3975, 2022, doi: 10.1109/JBHI.2022.3172976.
S. U. Saeed et al., “Bi-parametric prostate MR image synthesis using pathology and sequence-conditioned stable diffusion,” pp. 814–828, 2023, DOI: http://arxiv.org/abs/2303.02094
M. J. J. Ghrabat, G. Ma, I. Y. Maolood, S. S. Alresheedi, and Z. A. Abduljabbar, “An effective image retrieval based on optimized genetic algorithm utilized a novel SVM-based convolutional neural network classifier,” Human-centric Comput. Inf. Sci., vol. 9, no. 1, 2019, doi: 10.1186/s13673-019-0191-8.
E. T. Khalid, M. Salah Khalefa, W. Yassen, and A. Adil Yassin, “Omicron virus emotions understanding system based on deep learning architecture,” J. Ambient Intell. Humaniz. Comput., vol. 14, no. 7, pp. 9497–9507, 2023, doi: 10.1007/s12652-023-04615-8.
X. Xing, C. Wang, H. Zhou, J. Zhang, Q. Yu, and D. Xu, “DiffSketcher: Text Guided Vector Sketch Synthesis through Latent Diffusion Models,” no. NeurIPS, 2023, [Online]. Available: http://arxiv.org/abs/2306.14685
Z. A. Abduljabbar et al., “Provably Secure and Fast Color Image Encryption Algorithm Based on S-Boxes and Hyperchaotic Map,” IEEE Access, vol. 10, no. February, pp. 26257–26270, 2022, doi: 10.1109/ACCESS.2022.3151174.
D. Podell et al., “SDXL: Improving Latent Diffusion Models for High-Resolution Image Synthesis,” 2023, [Online]. Available: http://arxiv.org/abs/2307.01952
K. J. Joseph et al., “Iterative Multi-granular Image Editing using Diffusion Models,” pp. 8107–8116, 2023, [Online]. Available: http://arxiv.org/abs/2309.00613
A. Vuong et al., “Language-driven Scene Synthesis using Multi-conditional Diffusion Model,” no. NeurIPS, 2023, [Online]. Available: http://arxiv.org/abs/2310.15948
J. Kim and H. Park, “Adaptive Latent Diffusion Model for 3D Medical Image to Image Translation: Multi-modal Magnetic Resonance Imaging Study,” 2023, [Online]. Available: http://arxiv.org/abs/2311.00265
M. A. Mohammed, M. A. Hussain, Z. A. Oraibi, Z. A. Abduljabbar, and V. O. Nyangaresi, “Secure Content Based Image Retrieval System Using Deep Learning,” Basrah Res. Sci., vol. 49.2, no. 2, pp. 94–111, 2023, doi: 10.56714/bjrs.49.2.9.
P. Friedrich, J. Wolleb, F. Bieder, A. Durrer, and P. C. Cattin, “WDM: 3D Wavelet Diffusion Models for High-Resolution Medical Image Synthesis,” 2024, [Online]. Available: http://arxiv.org/abs/2402.19043
A. S. Lundervold and A. Lundervold, “An overview of deep learning in medical imaging focusing on MRI,” Z. Med. Phys., vol. 29, no. 2, pp. 102–127, 2019, doi: 10.1016/j.zemedi.2018.11.002.
T. Karras, M. Aittala, T. Aila, and S. Laine, “Elucidating the Design Space of Diffusion-Based Generative Models,” Adv. Neural Inf. Process. Syst., vol. 35, no. NeurIPS, 2022.
E. Ntavelis, A. Siarohin, K. Olszewski, C. Wang, L. Van Gool, and S. Tulyakov, “AutoDecoding Latent 3D Diffusion Models,” no. NeurIPS, 2023, [Online]. Available: http://arxiv.org/abs/2307.05445
L. Yang et al., “Improving Diffusion-Based Image Synthesis with Context Prediction,” no. NeurIPS, pp. 1–21, 2024, [Online]. Available: http://arxiv.org/abs/2401.02015
Y. Li et al., “SnapFusion: Text-to-Image Diffusion Model on Mobile Devices within Two Seconds,” no. NeurIPS 2023, pp. 1–17, 2023, [Online]. Available: http://arxiv.org/abs/2306.00980
W. Wu et al., “DatasetDM: Synthesizing Data with Perception Annotations Using Diffusion Models,” no. NeurIPS, pp. 1–13, 2023, [Online]. Available: http://arxiv.org/abs/2308.06160
J. Ho, C. Saharia, W. Chan, D. J. Fleet, M. Norouzi, and T. Salimans, “Cascaded Diffusion Models for High Fidelity Image Generation,” Journal of Machine Learning Research, vol. 23, pp. 1–33, 2022.
A. Shmakov, K. Greif, M. Fenton, A. Ghosh, P. Baldi, and D. Whiteson, “End-To-End Latent Variational Diffusion Models for Inverse Problems in High Energy Physics,” no. NeurIPS, pp. 1–26, 2023, [Online]. Available: http://arxiv.org/abs/2305.10399
S. Pan et al., “Synthetic CT Generation from MRI using 3D Transformer-based Denoising Diffusion Model.
J. Cao, Z. Wang, H. Guo, H. Cheng, Q. Zhang, and R. Xu, “Spiking Denoising Diffusio Probabilistic Models.” [Online]. Available: https://github.com/AndyCao1125/SDDPM
Z. Lv et al., “Learning Semantic Person Image Generation by Region-Adaptive Normalization,” Proc. IEEE Comput. Soc. Conf. Comput. Vis. Pattern Recognit., vol. 3, pp. 10801–10810, 2021, doi: 10.1109/CVPR46437.2021.01066.
K. N. S. Behara, A. Bhaskar, and E. Chung, “Insights into geographical window based SSIM for comparison of OD matrices,” ATRF 2017 - Australas. Transp. Res. Forum 2017, Proc., no. November, pp. 1–6, 2017.
J. Ko, I. Kong, D. Park, and H. J. Kim, “Stochastic Conditional Diffusion Models for Robust Semantic Image Synthesis”.
T. M. R. I. Synthesis, Q. H. Nguyen, and T. Le, “Class Label Conditioning Diffusion Model for Robust Brain Class Label Conditioning Diffusion Model for Robust Brain Tumor MRI Synthesis,” pp. 0–11, 2023, doi: 10.36227/techrxiv.24243829.v1.
M. Antonelli et al., “The Medical Segmentation Decathlon,” Nat. Commun., vol. 13, no. 1, pp. 1–13, 2022, doi: 10.1038/s41467-022-30695-9.
M. J. J. Ghrabat, G. Ma, Z. A. Abduljabbar, M. A. Al Sibahee, and S. J. Jassim, “Greedy Learning of Deep Boltzmann Machine (GDBM)’s Variance and Search Algorithm for Efficient Image Retrieval,” IEEE Access, vol. 7, pp. 169142–169159, 2019, doi: 10.1109/ACCESS.2019.2948266.
Z. A. Abduljabbar et al., “SEPIM: Secure and efficient private image matching,” Appl. Sci., vol. 6, no. 8, 2016, doi: 10.3390/app6080213.
X. Dai et al., “Multimodal MRI synthesis using unified generative adversarial networks,” Med. Phys., vol. 47, no. 12, pp. 6343–6354, 2020, doi: 10.1002/mp.14539.
J. Wolleb, F. Bieder, R. Sandkühler, and P. C. Cattin, “Diffusion Models for Medical Anomaly Detection,” Lect. Notes Comput. Sci. (including Subser. Lect. Notes Artif. Intell. Lect. Notes Bioinformatics), vol. 13438 LNCS, pp. 35–45, 2022, doi: 10.1007/978-3-031-16452-1_4.
P. Dhariwal and A. Nichol, “Diffusion Models Beat GANs on Image Synthesis,” Adv. Neural Inf. Process. Syst., vol. 11, no. NeurIPS 2021, pp. 8780–8794, 2021.
Z. Dorjsembe, H.-K. Pao, S. Odonchimed, and F. Xiao, “Conditional Diffusion Models for Semantic 3D Medical Image Synthesis,” 2023, doi: 10.21227/3ej9-e459.
A. Kumar Bhunia et al., “Person Image Synthesis via Denoising Diffusion Model,” pp. 5968–5976, 2023, doi: 10.1109/cvpr52729.2023.00578.
J. Ho, A. Jain, and P. Abbeel, “Denoising diffusion probabilistic models,” Adv. Neural Inf. Process. Syst., vol. 2020-Decem, no. NeurIPS 2020, pp. 1–25, 2020.
W. Wang et al., “Semantic Image Synthesis via Diffusion Models,” 2022, [Online]. Available: http://arxiv.org/abs/2207.00050
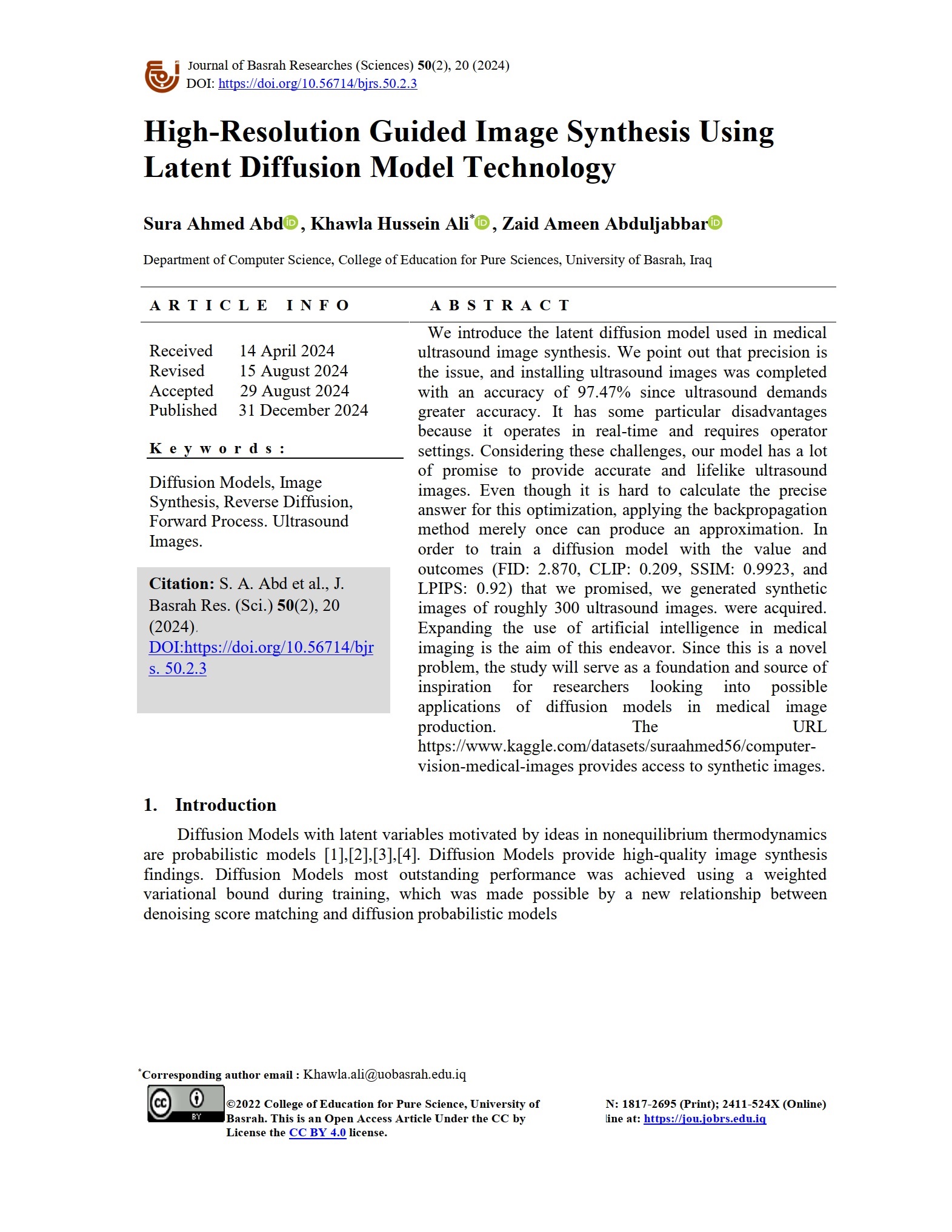
Downloads
Published
How to Cite
Issue
Section
License
Copyright (c) 2024 Basrah Researches Sciences

This work is licensed under a Creative Commons Attribution 4.0 International License.