Effectiveness of Artificial Intelligence in Mathematics Teaching by Protus 2.1
DOI:
https://doi.org/10.56714/bjrs.50.2.1Keywords:
Artificial Intelligence , mathematics education , Learning Styles , Protus .Abstract
The use of Artificial Intelligence (AI) in the field of mathematics education as a novel tool has introduced new capabilities in the learning and teaching processes. This technology not only assists teachers and students in enhancing the learning process but also provides the latest teaching methods.
One of the main advantages of AI in mathematics education is the ability to offer personalized learning. By analyzing individual data related to each student's learning style, AI systems can precisely adjust educational programs. This implies that each learner will follow a learning route that is suited to their knowledge level and needs. These technologies can help to diversify the learning process, catch students' interest, and boost their excitement for active engagement in the learning process. In this post, course content was personalized using the Protus 2.1 educational system.
Downloads
References
G. J. Hwang and Y. F. Tu, “Roles and research trends of artificial intelligence in mathematics education: a bibliometric mapping analysis and systematic review,” *Mathematics*, vol. 9, no. 6, p. 584, 2021.
R. Wu, “Visualization of basic mathematics teaching based on artificial intelligence,” in *Proceedings of the Journal of Physics: Conference Series*, I.O.P. Publishing, UK, August 2021.
M. A. Chaudhry and E. Kazim, “Artificial intelligence in Education (AIEd): a high-level academic and industry note 2021,” *AI and Ethics*, vol. 2, no. 1, pp. 157–165, 2022.
G. Malik, D. K. Tayal, and S. Vij, “An analysis of the role of artificial intelligence in education and teaching,” in *Recent Findings in Intelligent Computing Techniques*, pp. 407–417, Springer, Singapore, 2019.
A. Klanja-Milievi, B. Vesin, M. Ivanovi, and Z. Budimac, “E-Learning personalization based on hybrid recommendation strategy and learning style identification,” *Computers & Education*, vol. 56, pp. 885-899, April 2011.
B. Vesin, A. Klanja-Milievi, M.Ivanovi, and Z. Budimac, “Applying Recommender Systems and Adaptive Hypermedia for e-Learning Personalization,” *Computing and Information*, vol. 3, pp. 629-659, October 2013.
B. Vesin, M. Ivanovi, A. Klanja-Milievi, and Z. Budimac, "Protus 2.0: Ontology-based semantic recommendation in programming tutoring system," *Expert Systems with Applications*, vol. 39, no. 15, pp. 12229-12246, November 2012.
Moodle e-learning system, Available: https://moodle.com.
J.W. Keefe, “Learning style: An overview”, *Student learning styles: Diagnosing and prescribing programs*, pp. 1-17, 1979.
M. Grِnroos, *Book of Vaadin*, Lulu. com, 2011.
R. C. Clark & R. E. Mayer, *E-learning and the science of instruction: Proven guidelines for consumers and designers of multimedia learning*, John Wiley & Sons, 2016.
H.J. Yazici, "Role of learning style preferences and interactive response systems on student learning outcomes." *International Journal of Information and Operations Management Education*, vol. 6, no. 2, pp. 109-134, 2016.
Coffield, D. Moseley, E. Hall, and K. Ecclestone, *Learning styles and pedagogy in post learning: a systematic and critical review*, The Learning and Skills Research Centre, 2004.
J. Shen, and Q. Wu, “Application and cultivation on mathematical thinking in artificial intelligence,” in Proceedings of the 2021 4th International Conference on Intelligent Robotics and Control Engineering (IRCE), pp. 136–140, IEEE, Lanzhou, China, September 2021.
Q. Gao, H. Wang, F. Chang, H. Yi, and Y. Shi, “Reading achievement in China’s rural primary schools: a study of three provinces,” Educational Studies, vol. 47, no. 3, pp. 344–368, 2021.
E. Bahadir, “Using a neural network and logistic regression analysis to predict prospective mathematics teachers’ academic success upon entering graduate education,” Educational Sciences: 8eory and Practice, vol. 16, no. 3, pp. 943–964, 2016.
S. A. Al-Ghamdi, H. M. Mustafa, A. Al-Hamadi, M. H. Kortum, and A. MAl-Bassiouni, “On the optimality of teaching quality for a mathematical topic using neural networks (with a case study),” in Proceedings of the 2013 IEEE Global Engineering Education Conference (EDUCON), pp. 422–430, IEEE, Berlin, Germany, March 2013.
J. Stojanovic, D. Petkovic, I. M. Alarifi, et al., “Application of ´ distance learning in mathematics through adaptive neuro-fuzzy learning method,” Computers & Electrical Engineering, vol. 93, Article ID 107270, 2021.
S. Liu, T. He, and J. Dai, “A survey of CRF algorithm-based knowledge extraction of elementary mathematics in Chinese,” Mobile Networks and Applications, vol. 26, no. 5, pp. 1891– 1903, 2021.
M. Hooda, C. Rana, O. Dahiya, J. P. Shet, and B. K. Singh, “Integrating LA and E.D.M for improving Student’s Success in Higher Education using the F.C.N algorithm,” Mathematical Problems in Engineering, vol. 2022.
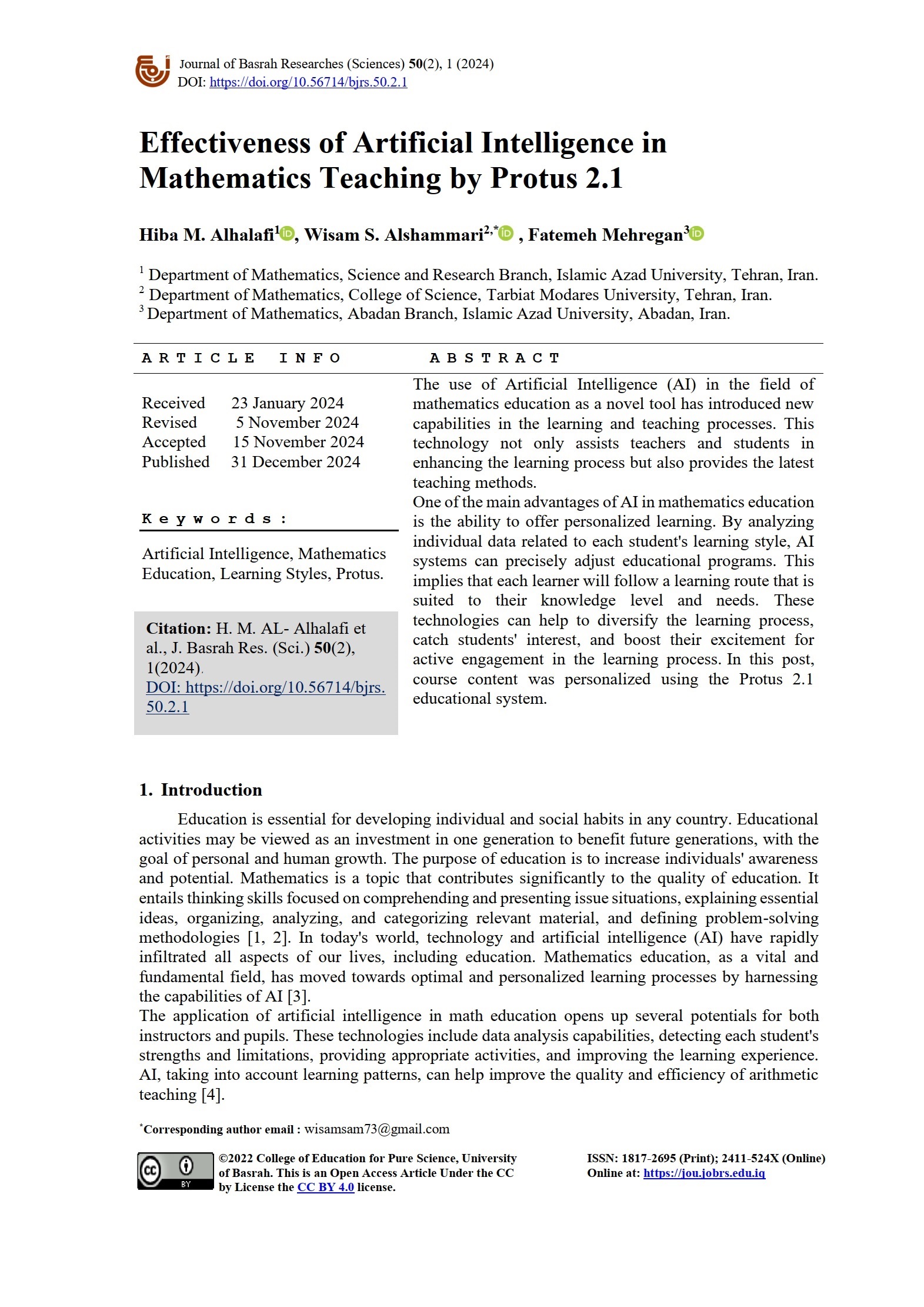
Downloads
Published
How to Cite
Issue
Section
License
Copyright (c) 2024 Basrah Researches Sciences

This work is licensed under a Creative Commons Attribution 4.0 International License.